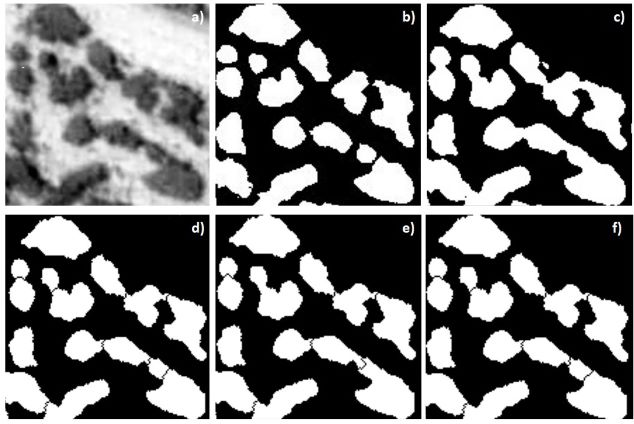
Refining Microstructural Analysis in Metallurgy: Semantic Segmentation Enhanced by Post-Processing
Abstract
This study investigates post-processing strategies to improve semantic segmentation models for microstructural characterization in metallurgy. Microstructural analysis is critical for understanding material properties and relies largely on expert knowledge. Deep learning algorithms have the potential for automation, but they encounter limitations such as small datasets and poor image quality. A watershed segmentation method is proposed after semantic segmentation, with a focus on the hypoeutectic Al-Si alloy. Post-processing methods were evaluated by automatically measuring the number of contours and average object area (features that could be fundamental in many microstructural images) within the study. After evaluating Euclidean, City Block, and Chebyshev distance transforms, the results demonstrate that while semantic segmentation provides high pixel accuracy, watershed segmentation enhances precision, particularly with City Block distance. Post-processed models have closer alignment with ground truth contours, indicating better segmentation. This emphasizes the importance of post-processing in improving semantic segmentation for metallurgical microstructural analysis, which allows for faster and more exact material assessments.
Full Text:
PDFReferences
F. Jiang, A. Grigorev, S. Rho, Z. Tian, Y. Fu, W. Jifara, K. Adil, S. Liu, Medical image semantic segmentation based on deep learning, Neural Comput. Appl. 29 (2018) 1257–1265.
H. Hwang, S.M. Choi, J. Oh, S.-M. Bae, J.-H. Lee, J.-P. Ahn, J.-O. Lee, K.-S. An, Y. Yoon, J.-H. Hwang, Integrated application of semantic segmentation-assisted deep learning to quantitative multi-phased microstructural analysis in composite materials: Case study of cathode composite materials of solid oxide fuel cells, J. Power Sources. 471 (2020) 228458.
J. Fu, S. Qu, J. Ding, X. Song, M.W. Fu, Comparison of the microstructure, mechanical properties and distortion of stainless steel 316 L fabricated by micro and conventional laser powder bed fusion, Addit. Manuf. 44 (2021) 102067.
W.R. Osório, L.C. Peixoto, M. V Canté, A. Garcia, Microstructure features affecting mechanical properties and corrosion behavior of a hypoeutectic Al–Ni alloy, Mater. Des. 31 (2010) 4485–4489.
S.M. Azimi, D. Britz, M. Engstler, M. Fritz, F. Mücklich, Advanced steel microstructural classification by deep learning methods, Sci. Rep. 8 (2018) 2128.
C. Herriott, A.D. Spear, Predicting microstructure-dependent mechanical properties in additively manufactured metals with machine-and deep-learning methods, Comput. Mater. Sci. 175 (2020) 109599.
M. Warmuzek, M. Żelawski, T. Jałocha, Application of the convolutional neural network for recognition of the metal alloys microstructure constituents based on their morphological characteristics, Comput. Mater. Sci. 199 (2021) 110722.
B.L. DeCost, T. Francis, E.A. Holm, Exploring the microstructure manifold: image texture representations applied to ultrahigh carbon steel microstructures, Acta Mater. 133 (2017) 30–40.
J. Jung, J. Na, H.K. Park, J.M. Park, G. Kim, S. Lee, H.S. Kim, Super-resolving material microstructure image via deep learning for microstructure characterization and mechanical behavior analysis, Npj Comput. Mater. 7 (2021) 96.
P. Warren, N. Raju, A. Prasad, M.S. Hossain, R. Subramanian, J. Kapat, N. Manjooran, R. Ghosh, Grain and grain boundary segmentation using machine learning with real and generated datasets, Comput. Mater. Sci. 233 (2024) 112739.
G. Fotos, A. Campbell, P. Murray, E. Yakushina, Deep learning enhanced Watershed for microstructural analysis using a boundary class semantic segmentation, J. Mater. Sci. 58 (2023) 14390–14410.
G. Zhu, R. Wang, Y. Liu, Z. Zhu, C. Gao, L. Liu, N. Sang, An adaptive post-processing network with the global-local aggregation for semantic segmentation, IEEE Trans. Circuits Syst. Video Technol. (2023).
D. Kochanov, F.K. Nejadasl, O. Booij, Kprnet: Improving projection-based lidar semantic segmentation, ArXiv Prepr. ArXiv2007.12668. (2020).
X. Cheng, H. Liu, A novel post-processing method based on a weighted composite filter for enhancing semantic segmentation results, Sensors. 20 (2020) 5500.
R. Ratajczak, C. Crispim, B. Fervers, E. Faure, L. Tougne, Semantic segmentation post-processing with colorized pairwise potentials and deep edges, in: 2020 Tenth Int. Conf. Image Process. Theory, Tools Appl., IEEE, 2020: pp. 1–6.
R. Usamentiaga, D.G. Lema, O.D. Pedrayes, D.F. Garcia, Automated surface defect detection in metals: A comparative review of object detection and semantic segmentation using deep learning, IEEE Trans. Ind. Appl. 58 (2022) 4203–4213.
G. Roberts, S.Y. Haile, R. Sainju, D.J. Edwards, B. Hutchinson, Y. Zhu, Deep learning for semantic segmentation of defects in advanced STEM images of steels, Sci. Rep. 9 (2019) 12744.
M.R.S. Ganesh, N. Reghunath, M. J. Levin, A. Prasad, S. Doondi, K. V Shankar, Strontium in Al–Si–Mg alloy: a review, Met. Mater. Int. (2022) 1–40.
J. Long, E. Shelhamer, T. Darrell, Fully convolutional networks for semantic segmentation, in: Proc. IEEE Conf. Comput. Vis. Pattern Recognit., 2015: pp. 3431–3440.
O. Ronneberger, P. Fischer, T. Brox, U-net: Convolutional networks for biomedical image segmentation, in: Med. Image Comput. Comput. Interv. 2015 18th Int. Conf. Munich, Ger. Oct. 5-9, 2015, Proceedings, Part III 18, Springer, 2015: pp. 234–241.
L.-C. Chen, G. Papandreou, I. Kokkinos, K. Murphy, A.L. Yuille, Deeplab: Semantic image segmentation with deep convolutional nets, atrous convolution, and fully connected crfs, IEEE Trans. Pattern Anal. Mach. Intell. 40 (2017) 834–848.
A. Chaurasia, E. Culurciello, Linknet: Exploiting encoder representations for efficient semantic segmentation, in: 2017 IEEE Vis. Commun. Image Process., IEEE, 2017: pp. 1–4.
M. Zhang, J. Wang, B. Wang, C. Xue, X. Liu, Quantifying the effects of Sc and Ag on the microstructure and mechanical properties of Al–Cu alloys, Mater. Sci. Eng. A. 831 (2022) 142355.
Copyright (c) 2024 Turkish Journal of Materials

This work is licensed under a Creative Commons Attribution-NonCommercial 4.0 International License.
Indexing: